Swipe right or swipe left? The seemingly simple act of choosing a potential partner on a dating app belies a complex world of algorithms. These sophisticated programs, often unseen by the user, dictate who we see, who we connect with, and ultimately, who we date. Understanding how these US dating app algorithms function is crucial to navigating the modern dating landscape and recognizing their potential impact on our romantic lives.
From the data points collected – location, age, interests, and even the time of day you’re active – to the diverse matching algorithms employed, such as collaborative filtering and content-based filtering, the inner workings of these apps shape our dating experiences profoundly. This exploration delves into the mechanics of these algorithms, examining their influence on user satisfaction, ethical considerations, and future trends.
How US Dating App Algorithms Function
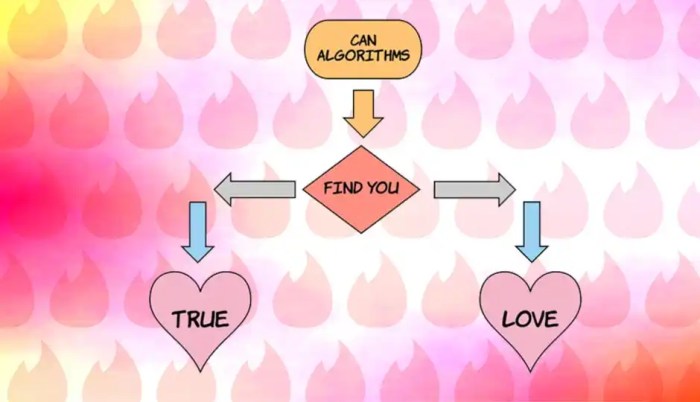
Source: digit.in
US dating app algorithms are complex systems designed to connect users based on shared preferences and compatibility. They leverage vast amounts of user data and sophisticated matching techniques to increase the likelihood of successful matches. Understanding how these algorithms work can provide insight into the user experience and the overall effectiveness of these platforms.
Core Mechanics of Dating App Algorithms
At their core, dating app algorithms work by analyzing user profiles and preferences to identify potential matches. This involves collecting and processing various data points, applying matching algorithms, and presenting users with a curated selection of potential partners. The process is iterative, constantly learning and refining its recommendations based on user interactions. The ultimate goal is to maximize user engagement and ultimately, successful relationships.
Data Points Used in Matching
Dating apps collect a wide array of data to build user profiles and inform matching suggestions. This data can be broadly categorized into:
- Demographic Information: Age, gender, location (often using GPS coordinates for precise location-based matching), and sometimes even education level and occupation.
- Profile Information: Self-described interests, hobbies, lifestyle preferences, and relationship goals (e.g., casual dating, serious relationship, marriage). This information is typically provided by the user through profile creation and questionnaires.
- Behavioral Data: This includes how users interact with the app, such as swiping patterns, messaging frequency, and the types of profiles they engage with. This data is crucial for understanding user preferences and refining matching algorithms over time.
- Photo Data: Image analysis techniques may be employed to assess the quality and attractiveness of user photos, although the ethical implications of this are increasingly being debated.
Matching Algorithms Employed
Several matching algorithms are used, often in combination, to generate potential matches. Two common approaches are:
- Collaborative Filtering: This algorithm identifies matches based on the preferences of similar users. If User A likes profiles similar to those liked by User B, the algorithm suggests User B as a potential match for User A. This method relies heavily on the collective behavior of the user base.
- Content-Based Filtering: This algorithm focuses on matching users based on the similarity of their profile information. If User A and User B have similar interests, hobbies, or relationship goals, the algorithm suggests them as a potential match. This approach is more direct, relying less on the actions of other users.
More sophisticated algorithms often combine these techniques, incorporating machine learning to improve accuracy and personalization over time.
Comparison of Dating App Algorithms
App | Algorithm | Data Points | Matching Criteria |
---|---|---|---|
Tinder | Hybrid (Collaborative & Content-Based Filtering, Machine Learning) | Age, Gender, Location, Photos, Interests (limited), Swipe Behavior | Proximity, Attractiveness (implicitly via photo analysis), Shared Interests (limited) |
Bumble | Hybrid (Collaborative & Content-Based Filtering, Machine Learning) | Age, Gender, Location, Photos, Interests, Education, Profession, Bio | Proximity, Shared Interests, Compatibility based on profile information |
Hinge | Hybrid (Collaborative & Content-Based Filtering, Machine Learning), emphasizes prompts and detailed profiles | Age, Gender, Location, Photos, Detailed Profile Information, Prompts, Connections | Shared Interests, Compatibility based on detailed profile and prompt responses, mutual connections |
Impact of Algorithms on User Experience
Dating app algorithms significantly influence user satisfaction and engagement. Their design directly impacts how users perceive the app, influencing everything from the initial sign-up experience to long-term retention. A well-designed algorithm can foster a positive and rewarding experience, leading to increased usage and positive word-of-mouth. Conversely, a poorly designed or biased algorithm can lead to frustration, a sense of unfairness, and ultimately, user churn.
The core functionality of a dating app hinges on its ability to connect compatible users. Algorithms determine which profiles are shown to each user, influencing the pool of potential matches and the overall experience. Factors like location, age, interests, and even communication style are considered, creating a personalized feed. However, this personalization can be a double-edged sword.
Algorithmic Influence on User Satisfaction and Engagement
Effective algorithms increase user engagement by presenting relevant matches and fostering meaningful interactions. Users who find compatible partners quickly are more likely to remain active on the app, leading to higher satisfaction and a positive feedback loop. Conversely, algorithms that consistently fail to deliver relevant matches can lead to user frustration and app abandonment. For example, an algorithm that prioritizes users based solely on superficial characteristics (e.g., physical attractiveness) might neglect important compatibility factors, resulting in a negative user experience and lower engagement rates. Conversely, an algorithm prioritizing shared interests and values can improve the likelihood of meaningful connections, boosting satisfaction.
Examples of Positive and Negative User Experiences
Positive user experiences are characterized by a steady stream of relevant profile suggestions, efficient communication tools, and a sense of progress toward the user’s dating goals. For instance, an app that successfully connects users with shared hobbies or life aspirations fosters a sense of accomplishment and boosts user satisfaction. A negative experience might involve being repeatedly shown incompatible profiles, experiencing technical glitches that hinder communication, or encountering profiles that violate the app’s terms of service. This can lead to a sense of wasted time and frustration, driving users away. A particularly frustrating experience might be being shown profiles that are geographically unrealistic given the user’s specified location parameters.
Algorithmic Bias and its Consequences
Algorithmic bias can significantly impact user experience, leading to unfair or discriminatory outcomes. For example, if an algorithm prioritizes certain demographic groups (e.g., younger, more attractive users), it might marginalize others, leading to feelings of exclusion and dissatisfaction. This bias can manifest in various ways, from the profiles displayed to the matching suggestions generated. The consequences of such bias can be severe, leading to a less inclusive and potentially harmful user experience. Research has shown that some algorithms inadvertently perpetuate existing societal biases, reinforcing inequalities in the online dating landscape.
Strategies for Improving User Experience Based on Algorithm Design
Improving the user experience requires a multifaceted approach to algorithm design. It’s crucial to move beyond simple matching based on superficial characteristics and incorporate more nuanced factors.
- Prioritize Transparency and Control: Users should have greater control over the algorithm’s parameters, allowing them to adjust preferences and filter options to align with their specific needs and desires. Transparency about how the algorithm functions helps build trust and empowers users.
- Implement Robust Bias Mitigation Techniques: Proactive measures should be taken to identify and mitigate potential biases in the algorithm’s design. Regular audits and testing can help ensure fairness and prevent discrimination.
- Incorporate User Feedback Mechanisms: Collecting user feedback through surveys, ratings, and reporting mechanisms allows for continuous improvement and refinement of the algorithm based on real-world experiences. This iterative process helps tailor the algorithm to user needs and preferences.
Ethical Considerations and Future Trends
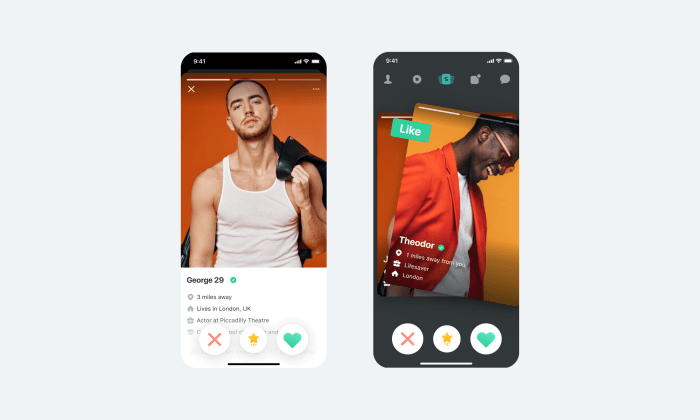
Source: datocms-assets.com
The increasing sophistication of US dating app algorithms raises significant ethical concerns, primarily revolving around data privacy, algorithmic transparency, and the societal impact of these powerful tools shaping romantic relationships. Understanding these concerns is crucial for responsible development and regulation of this rapidly evolving technology.
The core issue lies in the vast amounts of personal data collected by these apps. This data, often including location, relationship history, communication styles, and even personality assessments, is used to create user profiles and generate matches. The potential for misuse or unauthorized access to this sensitive information is considerable. Further, the lack of transparency regarding how these algorithms function creates a “black box” effect, making it difficult for users to understand how their data is used and how their matches are determined. This opacity undermines user agency and trust.
Data Privacy Concerns and Algorithmic Transparency
Dating apps employ diverse strategies for data collection and usage. Some apps are more transparent about their data practices, providing detailed privacy policies and allowing users to control the type of data collected and shared. Others operate with less transparency, leading to concerns about the potential for data breaches and the unauthorized sale or use of user data. For example, some apps might share anonymized data with third-party researchers, while others may utilize user data for targeted advertising, potentially blurring the lines between dating and commercial exploitation. The lack of consistent regulation across the industry exacerbates these issues.
Comparative Approaches to User Data Handling
A comparison of Tinder and Hinge illustrates varying approaches to data handling. While both collect extensive user data, Hinge emphasizes user control over profile information and data sharing, providing clearer explanations of its algorithmic processes. Tinder, on the other hand, has faced criticism for its less transparent data practices and its history of data breaches. This highlights the need for greater standardization and regulatory oversight in the industry to ensure user rights are protected. Furthermore, the growing use of AI in matching algorithms introduces new ethical dilemmas, as the basis for matching decisions becomes increasingly opaque.
Future Developments in Dating App Algorithms
Future developments are likely to see even more sophisticated AI-powered personality matching, building upon existing techniques. This could involve more nuanced analysis of communication styles, preferences, and values to create more compatible matches. Increased personalization will also be a key trend, with algorithms tailoring the user experience based on individual preferences and behavior. For instance, imagine an app that dynamically adjusts its matching algorithm based on a user’s feedback, leading to improved match quality over time. This approach, while potentially beneficial, also raises concerns about filter bubbles and the potential for reinforcing existing biases within the algorithm.
Societal Impact of Sophisticated Dating Algorithms
The societal impact of increasingly sophisticated dating app algorithms is multifaceted. On one hand, these algorithms can improve the efficiency of finding compatible partners, potentially leading to more fulfilling relationships. On the other hand, they can contribute to a homogenization of dating preferences and reinforce existing societal biases, such as racial or socioeconomic biases. The potential for algorithmic bias to perpetuate inequality and limit dating options for certain groups is a significant concern that requires careful consideration and mitigation strategies. The development of ethical guidelines and regulatory frameworks is crucial to ensure that these powerful tools are used responsibly and equitably.